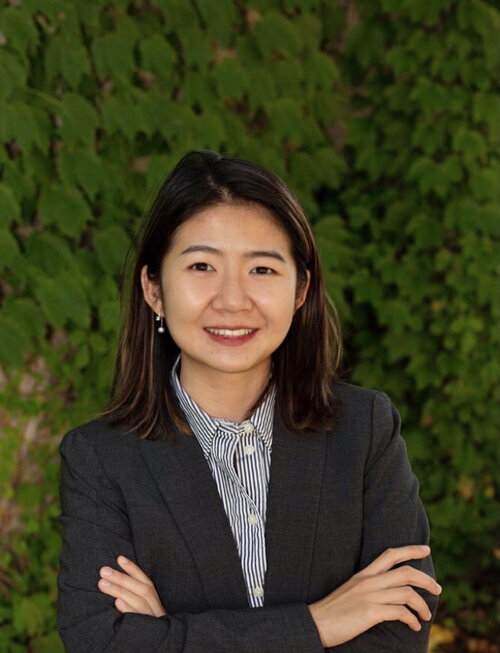
Research Interests
My research focuses on Bayesian theory and methodology, and their intersection with deep learning. In particular, my recent work examines simulation-based inference with generative AI models.
Education
Econometrics and Statistics, PhD, University of Chicago Booth School of Business
External Links
Recent Publications
Wang, Y., Polson, N., & Sokolov, V. O. (2023). Data Augmentation for Bayesian Deep Learning. Bayesian Analysis, 18(4), 1041-1069. https://doi.org/10.1214/22-BA1331
Wang, Y., Kaji, T., & Rockova, V. (2022). Approximate Bayesian Computation via Classification. Journal of Machine Learning Research, 23, Article 350.
Liu, Y., Ročková, V., & Wang, Y. (2021). Variable selection with ABC Bayesian forests. Journal of the Royal Statistical Society. Series B: Statistical Methodology, 83(3), 453-481. https://doi.org/10.1111/rssb.12423
Wang, Y., & Ročková, V. (2020). Uncertainty Quantification for Sparse Deep Learning. Proceedings of Machine Learning Research, 108, 298-308.